How data fusion can improve crop insurance
Role of modern crop insurance in agriculture and its challenges
The agriculture sector is a cornerstone of numerous economies worldwide, significantly contributing to food security and employment. However, it is intrinsically susceptible to a myriad of adversities including climatic anomalies, pests, diseases, and market fluctuations. Crop insurance emerges as a quintessential mechanism to cushion farmers against financial distress triggered by crop loss or damage. It acts as a safety net, ensuring that farmers can continue to operate post calamities, and thus holds a pivotal role in promoting agricultural sustainability and food security. Despite its importance, the efficacy of crop insurance largely hinges on accurate and timely assessment of crop damage, which remains a challenging endeavor. Traditional indemnity insurance assessment methods often entail on-field inspections and subjective evaluations, which are labor-intensive, time-consuming, and prone to inaccuracies. The alternative type of insurance – index or parametric insurance – allows for avoiding significant expenses and labor costs. Despite the fact that parametric insurance seems simpler and less expensive (sometimes), it also has disadvantages. The first issue is basic risk often correlated spatial and design risks. Often these risks are coming due to wrong or pure correlation of index/parameter with actual situation on the concrete field. The reasons for such situations may be the poor density of weather stations, misinterpretation of vegetation indices, gaps in the insured spatial coverage, etc. Sometimes insurers shall wait for confirmation of disasters from government institutions and it prolongs the time of payouts significantly.
What is data fusion?
The advent of remote sensing technology, drones, and ground-based sensors offers a plethora of data that can potentially revolutionize the domain of crop damage assessment for both types of insurance. These technologies can provide objective, high-resolution, and timely data depicting the state of crops over large expanses.
Data Fusion is a process of integrating multiple data sources to produce more consistent, accurate, and useful information about an entity than that provided by any individual data source. It is a multi-level process that handles the association, correlation, and combination of data and information from single or multiple sources to achieve refined position and identity estimates, and complete and timely assessments of situations and threats, and their significance.
Data fusion emerges as an innovative approach to harness the full potential of these disparate data sources. By amalgamating information from satellite imagery, drone footage, weather data, and on-ground sensors, data fusion can create a comprehensive, multi-dimensional view of the agricultural landscape. This fusion not only augments the spatial, temporal, and spectral resolutions of the data but also encapsulates a broader spectrum of information that can significantly enhance the accuracy and efficiency of crop damage assessment.
Why data fusion is important for crop insurance
Data fusion is crucial in crop insurance for several reasons which primarily revolve around enhancing accuracy, efficiency, and effectiveness in risk assessment, policy pricing, and claim processing. Here are some of the key reasons:
- Improving Risk Assessment: By fusing data from various sources such as satellite imagery, weather data, soil sensors, and historical claims data, insurers can obtain a more accurate understanding of the risks associated with insuring a particular crop or farm.
- Efficient Claim processing: Data fusion can speed up claims processing by automatically verifying claims using real-time and historical data. Our practice shows that using drones for claim adjusting speeds up data collection and processing 4-6 times in comparison to the manual procedure.
- Fraud Detection: Combining multiple data sources can help in identifying inconsistencies or anomalies in claims, which could be indicative of fraud.
- Address the Basis Risk for Index-based insurance products: fusion of various satellite and drone data allows to decrease the effects of basis risk for index insurance products.
- Continuous Learning and Improvement: The data collected and fused over time can be utilized to continuously improve risk models and operational processes, fostering a culture of data-driven decision-making and innovation Let me provide you with a number of obvious use cases in which data fusion works well.
For instance, after a reported weather event, satellite and drone imagery can be fused with weather data to quickly assess the damage and validate claims. Also, often it helps separate damages from one event from another (not claimed) and detect fraud. Fusion data from satellites and smartphones can help to detect and assess even the most complex cases connected with hail damage. Using Machine Learning for finding “patterns” among mixed satellite data, weather, drone-based images and photos from smartphones allows insurers to significantly reduce the operational costs associated with manual data collection and claims assessment. Additionally, accurate risk assessments and fraud detection can lead to lower claims payouts.
The usage of drones, led by satellite images allows to avoid extra payouts in the more expensive claims. A selective survey by drones of damaged areas detected by satellites helps to validate and clarify the initial assessment of damage. For those insurance companies, who’d committed and strongly believe in the fully manual claim adjustment, I can bring such news: on average, manual claim adjusting overestimates damage by 15-18%. Our practice shows that in fully remote damage assessment can give a precision of 5-8%. If mobile ground scouting is used, precision increases to 3-4%.
Professional opinion. Ian Shynkarenko, CEO of Agroinsurance.com says: “Loss adjustment cost for MPCI and other indemnity-based products may constitute between 5%-7% of the premium (GWP) with loss adjustment costs being less for wintering products and higher for spring-summer seasons. Claim accuracy may vary between the loss adjusters using same methods of calculation within 5%, which is normal in insurance industry. The leakage for crop claims due to the inaccuracies for estimation of damage areas and approximation of crop yields may run high up to 15-20%, which can certainly be addressed via a more accurate loss assessment with the use of remote sensing technologies and data fusion methods. The use of remote sensing can significantly reduce the time required for assessment of losses on field crops. So when looking at EO technologies, you may translate it into a potential 10-15% reduction of total loss assessment and indemnity payout costs. For crop insurance portfolios over 3 million EUR these efficiencies already show the need for EO data use. The savings on indemnity payouts raise the insurers profits, as wells provide available budgets for insurers to pay for deployment of good quality EO technologies in their daily underwriting and loss adjustment work.”
Data fusion methodology
Data fusion is a quite extensive field of knowledge, methods, tools, and frameworks. The whole process of data fusion included stages of
- data collection.
- data preprocessing (cleaning, normalization, and alignment of collected data).
- features extraction (Extraction of relevant features for crop damage assessment).
- data fusion itself (early, intermediate, and late fusion techniques used to integrate data).
- model development (various techniques can be employed depending on the nature of the data and the objectives, including statistical methods, probabilistic models, machine learning, deep learning, etc.).
- validation and testing of the model.
- optimization and deployment.
Also, you may provide data fusion itself on several levels. I provide below examples involving satellites and drone-based data:
- Data Level Fusion: merge satellite and drone imagery at the pixel level to create composite images.
- Feature Level Fusion: combine extracted features from both satellite and drone imagery.
- Decision Level Fusion: Utilize separate models (or processing tools) for satellite and drone imagery, and fuse the decision outputs.
Indeed, data fusion is not something standalone and self-sufficient. In most cases, it is an integrated and comprehensive process, that consists of several supportive steps for data processing and supporting decision-making.
On another side, you, as a decision-maker can do it as complex or simple as you want. It depends on how deeply you want to use math and AI in the process. Indeed, in some cases, you can limit yourself to minimal data processing and then compare the results on a level of general features. For example, if you get the vegetation index of the same field from satellites and drones, it would be enough to compare such features with the median or average index value and delve into the distribution of the index over the field.
Further, I provide you an example of a very practical comprehensive framework/tool, which along with many other functions, implements some basic elements of data fusion, including Machine Learning and Deep Learning incorporated engines. This pretty short and general introduction to the data processing capabilities of the system followed a valuable use case of using data fusion between satellite data and grons-based truth, collected from mobile devices.
Practical Data Fusion and ML framework
This chapter is based on the cloud geo-intelligence platform, provided by Skyglyph. Since 2016, skyglyph has been working in crop insurance with a cloud platform, which allows our customers to collect and analyze data from insured fields with the help of drones, precision satellites, and even smartphones and other IoT devices.
Integrating satellite-based technology and drones creates a powerful synergy for monitoring and analyzing agricultural areas in the context of an insurance program. Here’s how these technologies are integrated and leveraged together:
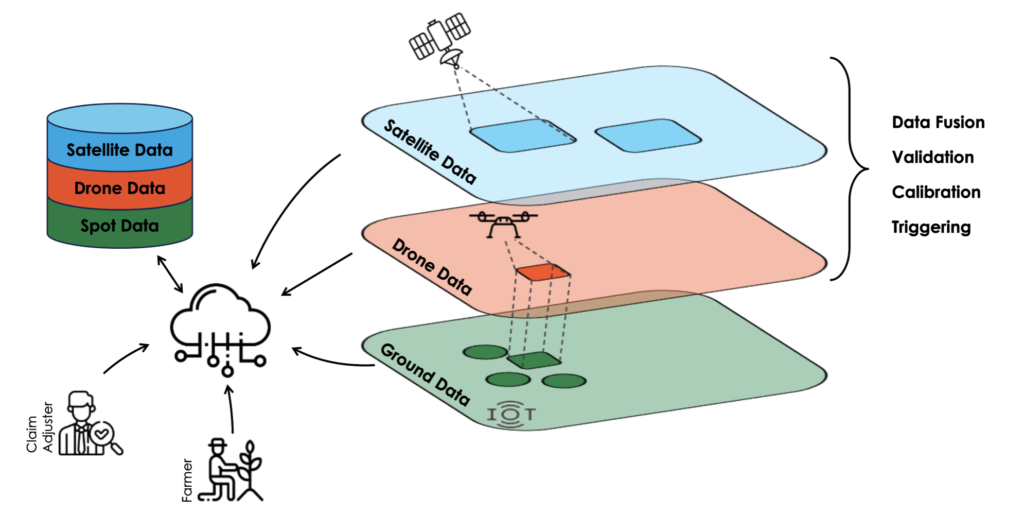
- Data Fusion allows to combination of satellite imagery with high-resolution drone imagery to create a more comprehensive and detailed view of agricultural lands.
- The calibration and Validation mechanism allows the use of drone data to improve the accuracy and reliability of satellite information.
- Triggering triggers drone inspections for further investigation in places where anomalies are detected by satellite. By integrating satellite-based technology and drones we significantly improve the ability of our system to assess risks, manage claims, and provide valuable insights for decision-making.
Use case: Assess the severity of damage with the help of fusion data from satellite and ground scouting
The next level of integration involves mobile technologies for the collection of visual and structural information from fields by POI (Points of Inspection) and then finding a correlation with satellite maps. In the example below, the correlation was found between several vegetation maps and a series of measured points- “severity of damage”:

As you see, the most relevant correlation is found with the satellite map from 30-06-2021. In our case, it was data on corn damage after a strong wind in the Balkan region. Now you can review the new “severity damage map”, built on the base of the correspondent sat map:
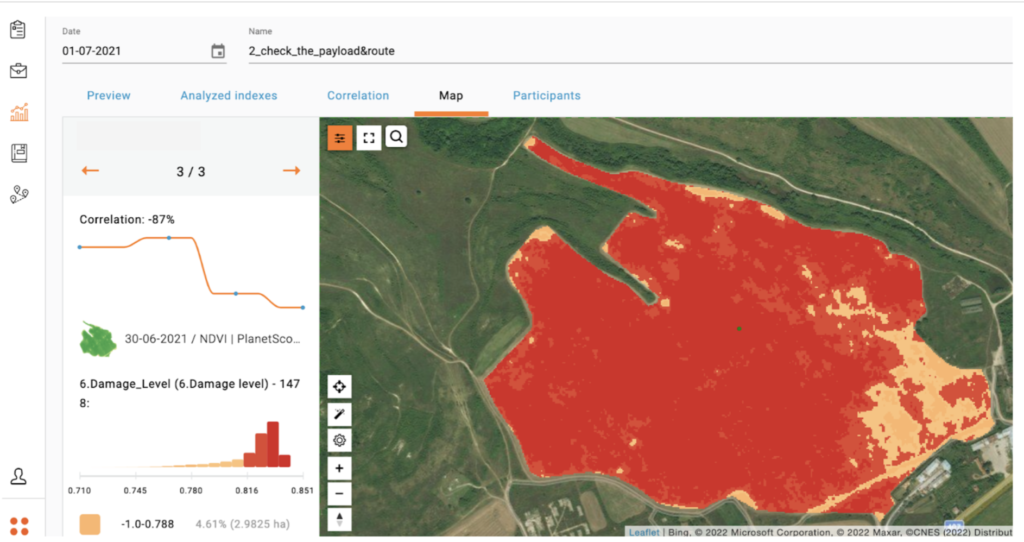
As a result, you can assess the level/severity of damage with the usage of ground truth information and simultaneously calibrate your satellite maps. Also, detailing of histogram allows you to estimate the percentage and acreage of damage:
Thus, only 14.14 ha (or 9.14%) is affected by the damage. The corresponding NDVI map confirms this information:
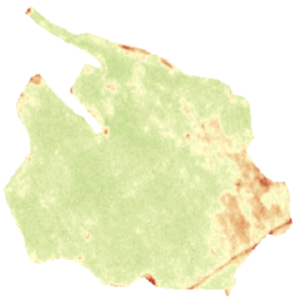
Summary
It’s evident that data fusion can play a transformative role in crop insurance. This approach not only addresses the limitations of traditional insurance models, such as issues with accuracy, efficiency, and risk assessment, but it also incorporates technological advancements, like drones, which can radically streamline processes including claim processing and fraud detection. The extent to which data fusion can be a game-changer in modernizing crop insurance depends largely on the ability of insurers to adopt and effectively integrate this technology into their core business operations.